Decision Tree to Classification of Dairy Cows from Genetic Information
DOI:
https://doi.org/10.36790/epistemus.v16i33.220Keywords:
Classification, Decision tree, Dairy productionAbstract
This paper presents decision trees as a machine learning technique for classifying cows as good milk producers or not, based on the use of genetic markers. The purpose is to select genetically superior animals in less time and make the assisted reproduction process more efficient, thereby reducing costs and increasing profits in the dairy sector. Results are presented on the efficiency of decision trees for the classification of dairy cows, up to 94.5% accuracy was achieved. In addition, the algorithm allowed the identification of the most dominant SNP for classification, and the chromosome that most influences the prediction.
Downloads
References
Cámara Nacional de Industriales de la Leche (CANILEC), “Estadísticas del sector lácteo 2010-2020,” 2021.
G. R. Wiggans, J. B. Cole, S. M. Hubbard, and T. S. Sonstegard, “Genomic Selection in Dairy Cattle: The USDA Experience∗,” Annu. Rev. Anim. Biosci., vol. 5, pp. 309–327, 2017. DOI: https://doi.org/10.1146/annurev-animal-021815-111422
C. E. Rabier, P. Barre, T. Asp, G. Charmet, and B. Mangin, “On the accuracy of genomic selection,” PLoS One, vol. 11, no. 6, pp. 1–23, 2016. DOI: https://doi.org/10.1371/journal.pone.0156086
B. Hayes and M. Goddard, “Genome-wide association and genomic selection in animal breeding,” Genome, vol. 53, no. 11, pp. 876–883, 2010. DOI: https://doi.org/10.1139/G10-076
M. E. Goddard and B. J. Hayes, “Genomic selection,” J. Anim. Breed. Genet., no. 124, pp. 323–330, 2007. DOI: https://doi.org/10.1111/j.1439-0388.2007.00702.x
B. Li, N. Zhang, Y. G. Wang, A. W. George, A. Reverter, and Y. Li, “Genomic prediction of breeding values using a subset of SNPs identified by three machine learning methods,” Front. Genet., vol. 9, no. JUL, pp. 1–20, 2018. DOI: https://doi.org/10.3389/fgene.2018.00237
M. I. Jordan and T. M. Mitchell, “Machine learning: Trends, perspectives, and prospects,” vol. 349, no. 6245, 2015. DOI: https://doi.org/10.1126/science.aaa8415
S. B. Kotsiantis, “Supervised Machine Learning: A Review of Classification Techniques,” Informatica, vol. 31, pp. 249–268, 2007.
T. Hastie, R. Tibshirani, and J. Friedman, “Unsupervised Learning,” in The Elements of Statistical Learning, 2009, pp. 486–585. DOI: https://doi.org/10.1007/978-0-387-84858-7_14
Z. Chen, Y. Yao, P. Ma, Q. Wang, and Y. Pan, “Haplotype-based genome-wide association study identifies loci and candidate genes for milk yield in Holsteins,” PLoS One, vol. 13, no. 2, pp. 1–13, 2018. DOI: https://doi.org/10.1371/journal.pone.0192695
M. A. Cleveland, J. M. Hickey, and S. Forni, “A common dataset for genomic analysis of livestock populations,” G3 Genes, Genomes, Genet., vol. 2, no. 4, pp. 429–435, 2012. DOI: https://doi.org/10.1534/g3.111.001453
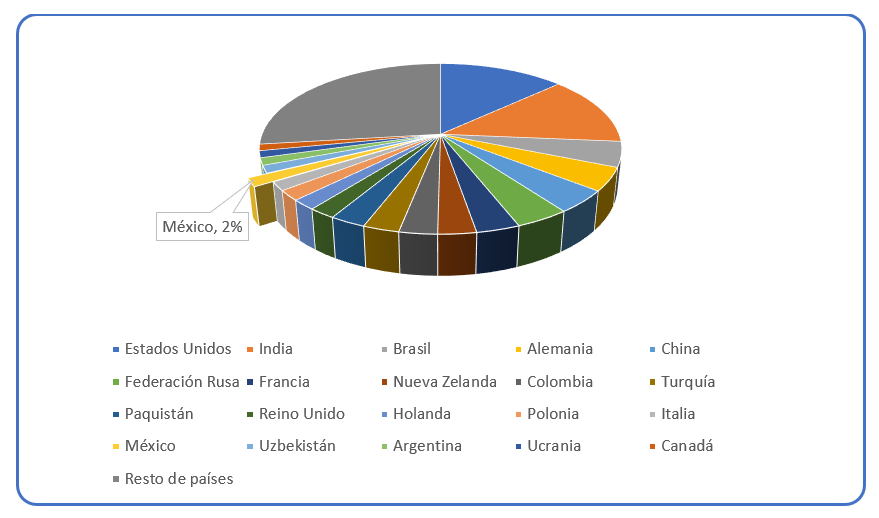
Downloads
Published
How to Cite
Issue
Section
License
Copyright (c) 2022 EPISTEMUS

This work is licensed under a Creative Commons Attribution-NonCommercial-ShareAlike 4.0 International License.
The magazine acquires the patrimonial rights of the articles only for diffusion without any purpose of profit, without diminishing the own rights of authorship.
The authors are the legitimate owners of the intellectual property rights of their respective articles, and in such quality, by sending their texts they express their desire to collaborate with the Epistemus Magazine, published biannually by the University of Sonora.
Therefore, freely, voluntarily and free of charge, once accepted the article for publication, they give their rights to the University of Sonora for the University of Sonora to edit, publish, distribute and make available through intranets, Internet or CD said work, without any limitation of form or time, as long as it is non-profit and with the express obligation to respect and mention the credit that corresponds to the authors in any use that is made of it.
It is understood that this authorization is not an assignment or transmission of any of your economic rights in favor of the said institution. The University of Sonora guarantees the right to reproduce the contribution by any means in which you are the author, subject to the credit being granted corresponding to the original publication of the contribution in Epistemus.
Unless otherwise indicated, all the contents of the electronic edition are distributed under a license for use and Creative Commons — Attribution-NonCommercial-ShareAlike 4.0 International — (CC BY-NC-SA 4.0) You can consult here the informative version and the legal text of the license. This circumstance must be expressly stated in this way when necessary.
The names and email addresses entered in this journal will be used exclusively for the purposes established in it and will not be provided to third parties or for their use for other purposes.